MEET research programs
MEET aims to conduct research to lead the world in realizing a networking structure that can transmit information beyond the physical limit of communication channel capacity, through the innovative networking structure called “Meta-Networking”. Based on the demand for various services and the characteristics of data, we will perform innovative and evolutionary distributed control among network entities to increase information density within the network. Instead of transmitting the source data as it is, we will extract and transmit only the meaningful parts for the recipient’s needs. Compared to the conventional simple transmission method of source data, which transmits the data as it is, we aim to increase the amount of information that can be transmitted by over 100 times (based on initial considerations for image data distribution) and achieve a significant improvement in networking performance. In Meta-Networking, the aim is to ensure that the “meaning” of the source data is correctly received. To achieve such a fundamental reform of the networking method, there are various challenging technical issues that need to be addressed.
How to characterize the QoE requirements of different services?
By analyzing the QoE requirements addressed by various types of services, including current applications and possible applications in the future, like human-collaborative robots and digital twins of real 3D space. Then classify the services into multiple types of QoE requirements, and design a general service characterization scheme to address different types of services.
How to extract the features of each service and the corresponding data?
Data generated in the application space are of all kinds, including text, image, video, and so on. To ensure effective feature extraction in all kinds of data, cutting edge AI technologies are needed to create feature extraction methods and optimizing feature retrieving process is necessary for supporting transmissions in the physical space.
How to exploit meta knowledge and extracted features for wireless access design to maximize information density while minimizing power consumption?
To fulfill this task, this research exploits the features and knowledge extracted by the above-mentioned tasks to design tailored radio and energy resource allocation as well as wireless access protocols. Wireless resource allocation and access protocols will be optimized from the joint perspectives of information density maximization and energy consumption minimization. AI technologies including Deep Learning and Reinforcement Learning, and advanced mathematical optimization and communication theory (such as finite block length transmission) are employed.
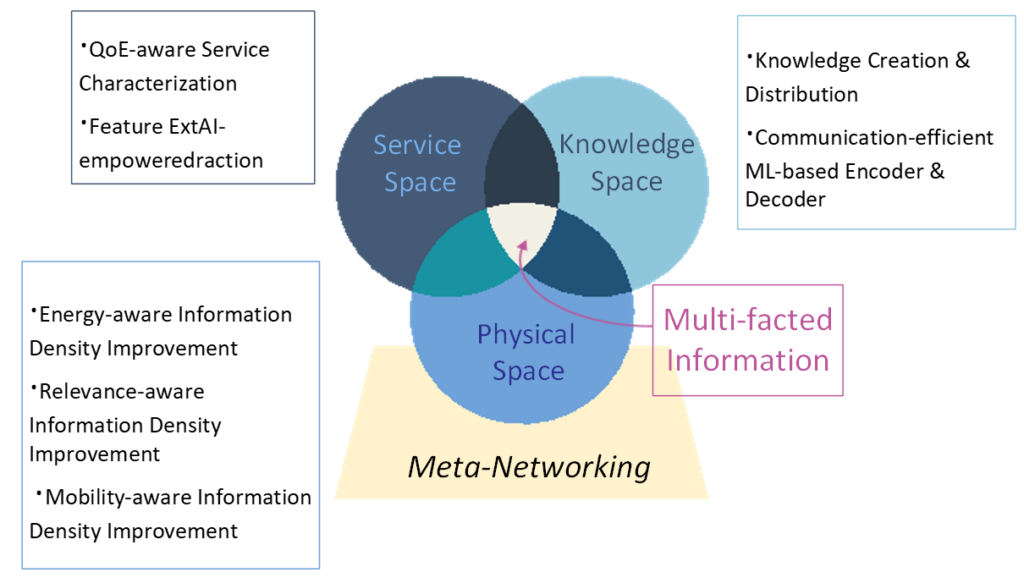
How to exploit the relevance between the service and information in the resource allocation to maximize information density?
To overcome the Shannon capacity limit of current wireless networks, this task explores relevance-aware information density improvement by means of joint optimization of different types of resources, including communication, computing, sensing, and caching resources with different levels of capabilities. Relevance-aware performance metrics are adopted such value of information, information freshness, and the accuracy of computation. Integrated computation and communication by means of over-the-air computation, and integrated sensing and communications by means of passive sensing using communication waveforms in heterogeneous network environments are also included.
How to manage and utilize mobility in various conditions to maximize information density?
Information density improvement and mobility, complement each other. “Moving resource” proposes an approach that estimates and pre-allocates the required resources and data to the vicinity of the user based on a proactive manner. The other way around, using the mobility of some network entities can improve the efficiency of task execution, where the incentive design of network entities for collaboration is also considered in the design based on the concept of Meta-networking.
How to create and distribute knowledge in a dynamic and distributed network?
Knowledge base modeling is quite important in further improving the information density. Deep reinforcement leaning-based approaches can distribute data in order to better support information density improvement in physical space for various services and conditions. The multi-faceted information are jointly considered in the knowledge distribution.
How to design and implement a communication-efficient encoder and decoder?
This task exploits federated learning and split computing and develops novel training and inference schemes enabling low-latency and highly-accurate training and inference without increasing communication traffic.